Technology
Quantum Machines and Nvidia are using machine learning to get closer to an error-correcting quantum computer
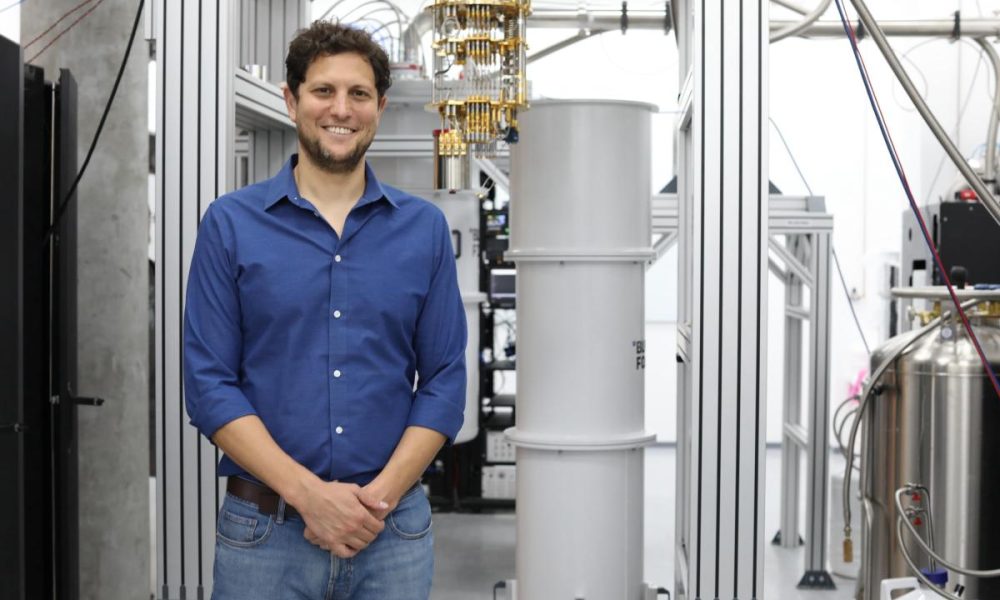
ABOUT a yr and a half agolaunch of quantum control Quantum machines and Nvidia announced a deep partnership that can bring together Nvidia firms DGX quantum computing platform and advanced quantum control equipment Quantum Machine. We have not heard much concerning the results of this collaboration for some time, nevertheless it’s now starting to bear fruit and bringing the industry one step closer to the holy grail of an error-correcting quantum computer.
Both firms demonstrated this during a presentation held earlier this yr can use a ready-made reinforcement learning model running on Nvidia’s DGX platform to higher control the qubits within the Rigetti quantum chip through system calibration.
Yonatan Cohen, co-founder and chief technology officer of Quantum Machines, noted that his company has long sought to use classical computing engines to control quantum processors. These compute engines were small and limited, but that is not an issue with Nvidia’s incredibly powerful DGX platform. The Holy Grail, he said, is using quantum error correction. We’re not there yet. Instead, this cooperation focused on calibration, and specifically on the calibration of the so-calledπ pulses” that control the rotation of the qubit contained in the quantum processor.
At first glance, calibration may appear to be a one-time problem: you calibrate the processor before you run the algorithm on it. But it is not that easy. “If you look at the performance of quantum computers today, you get high fidelity,” Cohen said. “But when users use a computer, it’s always not of the best quality. It’s consistently drifting. If we are able to recalibrate it steadily using these sorts of techniques and underlying hardware, we are able to improve performance and maintain (high) fidelity for a very long time, which shall be needed in quantum error correction.
Continuously adjusting these pulses in near real time is an extremely computationally intensive task, but because a quantum system is at all times barely different, additionally it is a control problem that will be solved using reinforcement learning.
“As quantum computers get bigger and better, there are all these problems that come up and become bottlenecks that require really a lot of computing power,” said Sam Stanwyck, product manager for Nvidia’s quantum computing group. “Quantum error correction is really huge. This is necessary to unlock error-tolerant quantum computing, but also how to apply exactly the right control pulses to get the most out of qubits.”
Stanwyck also emphasized that before DGX Quantum, there was no system that might achieve the minimum latency mandatory to perform these calculations.
As it seems, even small improvements in calibration can lead to huge improvements in error correction. “The return on investment in calibration in the context of quantum error correction is exponential,” explained Ramon Szmuk, product manager of Quantum Machines. “If you calibrate 10% better, you get exponentially better logic error (performance) in a logical qubit that is made up of many physical qubits. So we have a lot of motivation to calibrate very well and quickly.”
It is value emphasizing that this is just the start of the optimization and cooperation process. The team really just took a couple of off-the-shelf algorithms and saw which one worked best (TD3on this case). In total, the actual code to conduct the experiment was only about 150 lines long. Of course, this is dependent upon all of the work each teams have done to integrate the varied systems and construct the software stack. However, for developers, all this complexity will be hidden, and each firms expect to create more and more open source libraries over time to benefit from this larger platform.
Szmuk emphasized that on this project the team only worked with a really basic quantum circuit, but it will probably be generalized to deep circuits as well. If it will probably be done with one gate and one qubit, it will probably even be done with 100 qubits and 1,000 gates,” he said.
“I would say that an individual result is a small step, but it is a small step towards solving the most important problems,” Stanwyck added. “Useful quantum computing will require tight integration of accelerated supercomputing – and this may be the most difficult engineering challenge yet. So by being able to really do this on a quantum computer and tune the pulse in a way that’s optimized not just for a small quantum computer, but that is a scalable, modular platform, we think we’re really well on our way to solving some of the most important problems in quantum computing.”
Stanwyck also said the 2 firms plan to proceed this collaboration and bring these tools to more researchers. With Nvidia’s Blackwell chips arriving next yr, the corporate may have an much more powerful computing platform for this project as well.